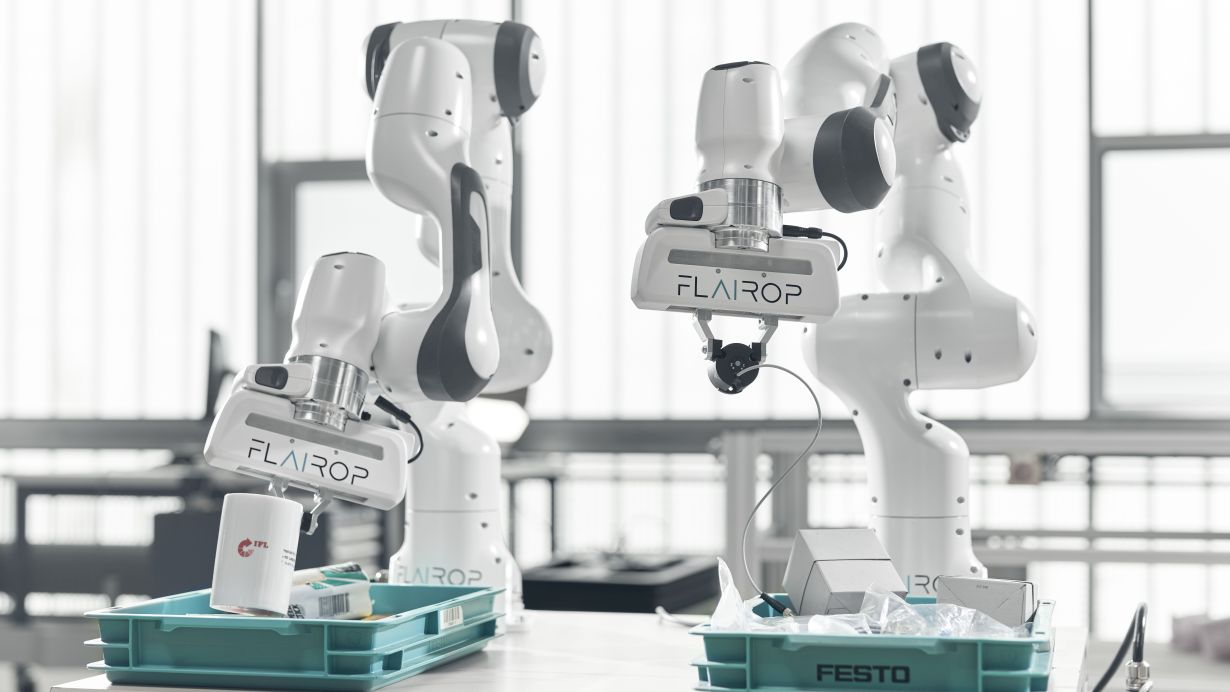
Researchers develop data-secure training platform for intelligent industrial robots.
Autonomous, flexible robots are considered a key technology for Industry and Logistics 4.0. The problem: In order to train robots controlled with artificial intelligence (AI), very large amounts of data are required, which only very few companies have. The solution: Robots from different companies at different locations learn from each other. Researchers at the Karlsruhe Institute of Technology (KIT) have worked with partners to develop ways of learning together without having to share sensitive data and trade secrets.
“With conventional machine learning methods, all data is collected and the AI is trained on a central server,” says Maximilian Gilles from the Institute for Materials Handling and Logistics Systems (IFL) at KIT. Through joint but locally separate learning, also known as federated learning, training data from several stations, from several plants or even several companies can be used without the participants having to disclose sensitive company data. “We have now been able to train autonomous gripping robots in logistics so that they are able to reliably grip items that they have not seen before,” says Gilles. Due to the variety of objects in an industrial warehouse, this is a very demanding task.
Training without central data collection
In the FLAIROP project, which started in 2021 and has now been completed, there was no exchange of data such as images or grip points for the training, but only the local parameters of the neural networks, i.e. highly abstracted knowledge, were transmitted to a central server. There, the weights from all stations were collected and combined using various algorithms. Then the improved version was pushed back to the local stations and further trained on the local data. This process was repeated several times. “Our results show that federated learning can be used to collaboratively create robust AI solutions for use in logistics without sharing sensitive data,” says Sascha Rank from the Institute for Applied Computer Science and Formal Description Methods (AIFB) at KIT was also a partner in FLAIROP.
In the future, the researchers want to further develop their federated learning system in such a way that, as a platform, it enables different companies to train robot systems together without having to share data with each other. Maximilian Gilles and his team are looking for partners from industry and research for further research.
A total of five autonomous picking stations were set up to train the robots: two at the IFL and three at Festo SE, based in Esslingen am Neckar. “We are pleased that we managed to show that robots can learn from each other without sharing sensitive data and trade secrets. This allows us to protect our customers’ data and we also gain speed because the robots can take on many tasks faster in this way. For example, the collaborative robots can support production workers with repetitive, difficult and tiring tasks,” says Dr. Jan Seyler, Head of Advanced Development Analytics and Control at Festo.
The research project FLAIROP
The FLAIROP project (stands for: Federated Learning for Robot Picking) was a partnership between Canadian and German organizations and companies. The Canadian project partners focused on object recognition through deep learning, explainable AI and optimization, while the German partners contributed their expertise in robotics, autonomous gripping through deep learning and data security.
- KIT-IFL: Development of an algorithm for determining the grasping point, development of automatic learning data generation
- KIT-AIFB: Development of Federated Learning Framework
- Festo SE und Co. KG: Consortium management, development of picking stations, piloting
- University of Waterloo (Canada): Development of an object recognition algorithm
- Darwin AI (Canada): Local and Global Network Optimization
FLAIROP was funded by the Canadian National Research Council (NRC) and the German Federal Ministry for Economic Affairs and Climate Protection (BMWK). Around 750,000 euros went to the KIT.
Photo: Amadeus Bramsiepe, KIT Karlsruhe Institute of Technology
Source: Karlsruhe Institute of Technology KIT, Aug 22, 2023
https://www.kit.edu/